Environmental Transformations and Interactions
Machine Learning Used to Analyze Molecules in Soil Organic Matter From Across the United States
Molecules from soil organic matter collected through the Molecular Observation Network enhances continental-scale understanding of soil microbial respiration.
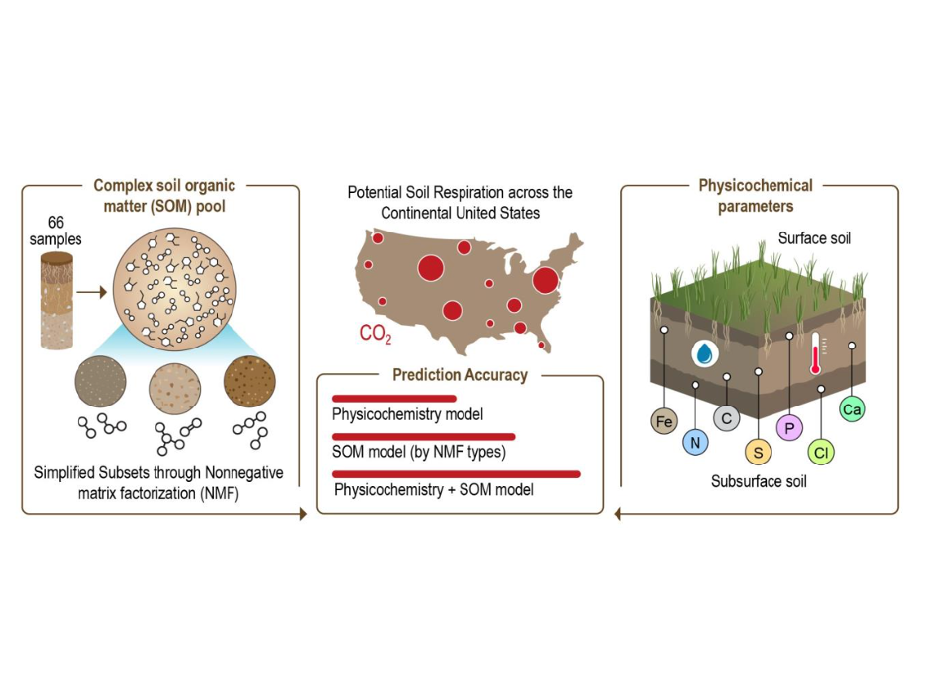
Machine learning extracts key molecules from complex high-resolution soil organic matter profiles to explain differences in potential soil respiration better than typically measured parameters. (Image courtesy of Nathan Johnson | Pacific Northwest National Laboratory)
The Science
Microbial respiration of soil organic matter (SOM) is a key contributor to the flux of carbon dioxide (CO2) from the soil and to the global carbon cycle. However, researchers do not fully understand this process, causing uncertainty in atmospheric predictions. Scientists have suggested that studying specific organic molecules in the soil could help, but initial efforts to do so have produced mixed results. In this study, a team of researchers led by the Environmental Molecular Sciences Laboratory (EMSL), a Department of Energy Office of Science user facility located at the Pacific Northwest National Laboratory, used machine learning (ML) to analyze detailed SOM data from across the United States. Using data from the Molecular Observation Network (MONet), an open science network developed by EMSL, the team found that the use of ML to interrogate SOM composition data could improve predictions of soil respiration, thereby enabling better prediction of how soils release carbon on a large scale.
The Impact
Molecular data has long held a promise for greater process-based understanding of soil carbon cycles, but using molecular information to substantially improve predictions of microbially-driven soil respiration has proved elusive. A major challenge in this effort is scaling the thousands of compounds found in SOM into tractable units for process-based models, a process made exponentially easier with current ML techniques. The approach developed in this study has provided a vital step in overcoming this scaling challenge by extracting subsets of molecules that improve statistical predictions of potential soil respiration across the continental United States. This outcome provides deeper understanding into the biogeochemistry of SOM decomposition and creates a strong basis for developing new model representations of soil carbon cycles.
Summary
Knowing how microbes break down SOM is important not only for understanding the flux of CO2 from soils, but the carbon cycle generally. Current models that predict soil carbon cycling mostly use atmospheric and soil property data, but these models have large uncertainty in their estimates due to the variety of factors that must be analyzed and considered. Researchers hypothesized that looking at the molecules in the soil might help improve these models. As part of this multi-institutional study, data from MONet was used to analyze the molecular composition of SOM from 66 soil samples from across the United States. The significant advancement in this research was the use of an ML model (NMFk) to simplify the analyses of the complex SOM found in each soil sample. The study clearly shows that understanding the molecular composition of SOM is important for predicting how soils release carbon. The authors suggest that this approach should be included in regional or local studies because it will enable modeling that could improve predictions of carbon cycling, critical for enhanced management of local or regional resources.
Contact
Emily Graham
Environmental Molecular Sciences Laboratory | Pacific Northwest National Laboratory
emily.graham@pnnl.gov
Funding
Soil data was provided by the Molecular Observation Network at the Environmental Molecular Sciences Laboratory, a Department of Energy Office of Science user facility sponsored by the Biological and Environmental Research program. Work was also conducted using capabilities available from the Joint Genome Institute, another DOE Office of Science user facility. Soil samples collected for the project were obtained through the National Ecological Observatory Network, a program sponsored by the National Science Foundation and operated under a cooperative agreement by Battelle.
Publication
S. Cheng, et al. “Scaling High-Resolution Soil Organic Matter Composition to Improve Predictions of Potential Soil Respiration Across the Continental United States,” Geophysical Research Letters 52, e2024GL113091 (2025). [DOI: 10.1029/2024GL113091]