Computing, Analytics, and Modeling
Functional and Systems Biology
Using Computation to Design Better Microbes
New quantum chemistry pipeline helps expedite microbial engineering by predicting how metabolic changes will affect modified organisms.
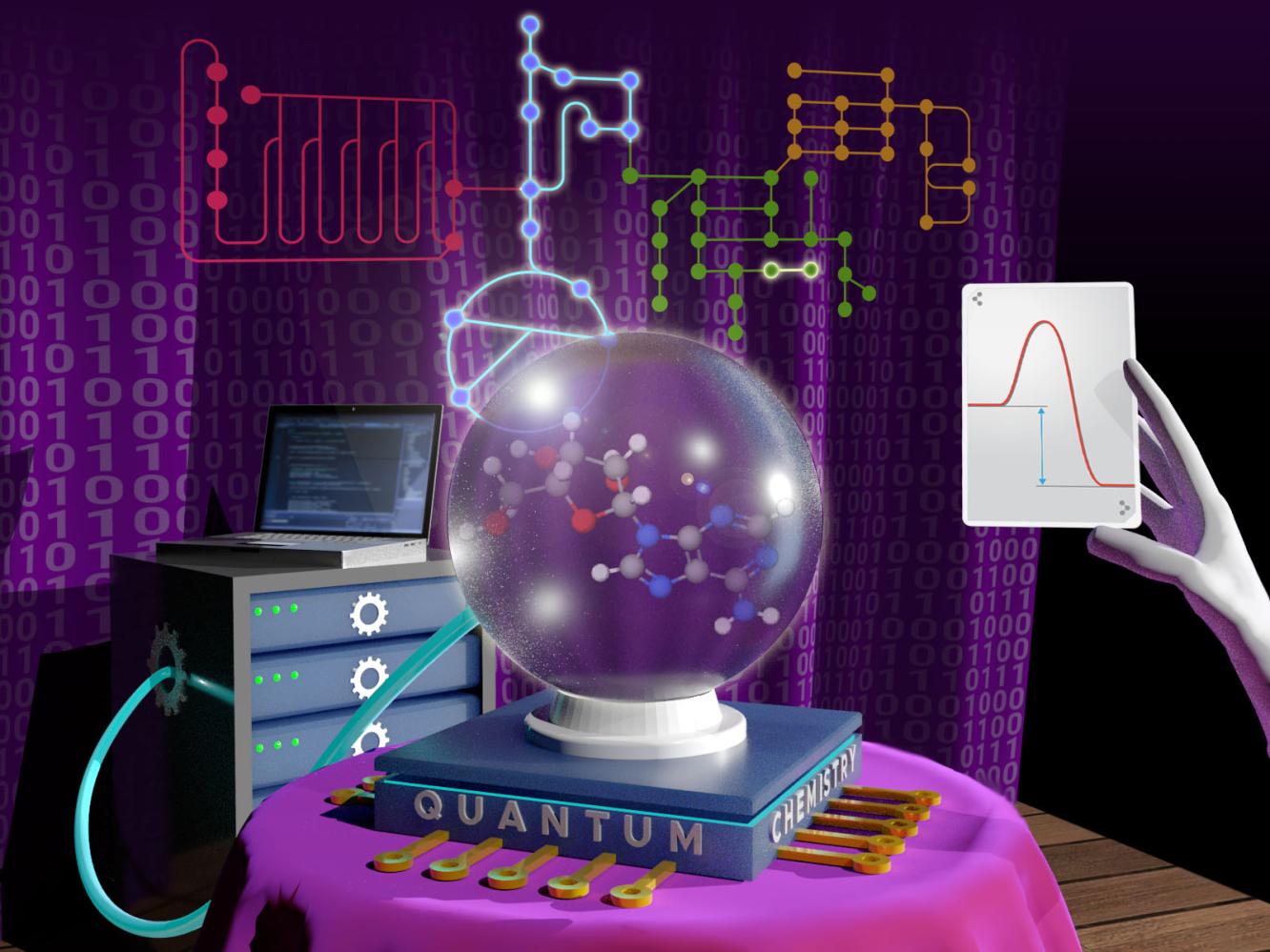
Despite their small size, microbes have a huge impact on human lives. People have exploited the power of microorganisms for several millennia, from making ancient wines and cheeses, to engineering them to produce next-generation biofuels and achieve better crop yields in modern times. Scientists have yet to unleash the full potential of microbes, as engineering them is an arduous task that typically requires many trial-and-error steps in the laboratory.
Imagine you are a researcher wanting to engineer a microbe to produce a specific biofuel. First, you might design a gene for a specialized protein called an enzyme that can synthesize said biofuel, then insert that gene into your organism of interest. If all goes well, your microbe will be able to successfully produce a working enzyme that can synthesize the biofuel. However, it is possible that your new gene or enzyme may negatively affect other metabolic pathways in the cell. If the effects were great enough, you would need to re-engineer the affected metabolic pathways of your organism to circumvent them. You would then repeat these laboratory-based approaches until your desired effects are achieved.
Research teams the world over are engaged in this kind of trial-and-error process to engineer microbes to do work for us. But what if there were a tool to streamline this process?
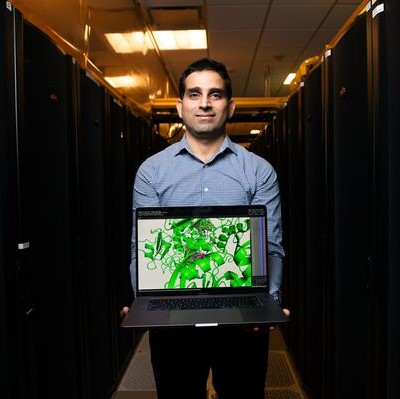
A team of researchers at Pacific Northwest National Laboratory and the Environmental Molecular Sciences Laboratory (EMSL), led by Neeraj Kumar, have now created a computational pipeline, called CompMol_Thermo, to predict how cells will be affected by metabolic changes. The results of this study were featured on the cover of ACS Omega.
Making predictions
A bacterial cell contains over a thousand different enzymes, each catalyzing—accelerating the rate of—a biochemical reaction, such as converting food into energy or synthesizing amino acids. Each reaction requires or produces a certain amount of energy, which can be quantified by thermodynamics. When scientists engineer bacteria to contain new enzymes and catalyze new reactions, the thermodynamics of other reaction pathways may be affected.
Kumar, along with collaborators from Argonne National Laboratory and Lawrence Berkeley National Laboratory, built an automated software pipeline capable of calculating the energetics of biochemical reactions and that very closely matches experimental values. This marks the first time a computational system has been able to replicate experimental thermodynamic values within the experimental error range. Researchers could use this process to design a microbe in silico and calculate the effects of their intended engineering. This information would be used to determine which changes would most likely produce the desired effects, and greatly reducing the trial-and-error process to only a small number of possibilities with a high likelihood of success.
Making quantum chemistry more user-friendly
EMSL is a U.S. Department of Energy Office of Science user facility where researchers can submit proposals to use EMSL facilities to support their own research. To ensure continuous capability growth, EMSL funds internal research, called Facility Research Investments, which includes support for computational development programs that bring users back to EMSL for their high-performance computing needs. When EMSL’s chief data and analytics officer, Lee Ann McCue, heard about Kumar’s idea to build a quantum chemistry pipeline, she knew she had something special on her hands.
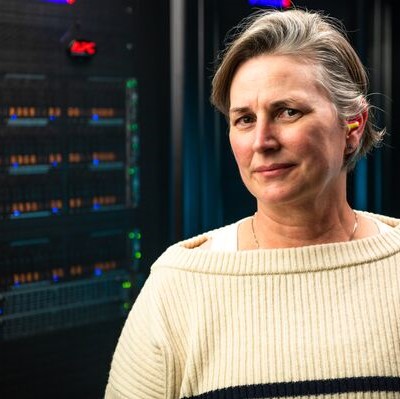
“I try to identify computational projects that can help scientists accelerate their research. This new pipeline has the potential to revolutionize the microbial engineering process by converting months or years of benchwork to hours of computation,” states McCue.
The thermodynamic computational modeling pipeline, called CompMol_Thermo, brings together software developed over several decades across three different national laboratories, merging two different funding streams from the Office of Science's Biological and Environmental Research program. It makes doing thermodynamic calculations more user-friendly by providing an automated system that takes inputs from the ModelSEED database, a metabolic modeling database spearheaded by Chris Henry from Argonne National Laboratory, and processes them for quantum chemical and molecular dynamics calculations by NWChem, an ab initio computational chemistry software developed by EMSL. The output by NWChem feeds back into ModelSEED to optimize the calculations. The pipeline and user narrative are available through KBase, the U.S. Department of Energy Systems Biology Knowledgebase.
The outputs of the CompMol_Thermo pipeline were validated through comparison to experimental data from the National Institute for Standards and Technology Thermodynamics of Enzyme-catalyzed Reactions database, which showed that the computational results were within the same margin of error as the experimental studies.
Looking ahead: EMSL in 2030
Kumar’s project is one of many at EMSL that reflects the facility’s goal of becoming the Modeling and Data Sciences hub for the Biological and Environmental Research program by 2030. EMSL’s decades of computing expertise, data analysis and visualization, multi-scale modeling, and molecular simulations provide a strong foundation to build upon.
By leveraging EMSL’s sophisticated software and new, mixed-architecture supercomputer, Tahoma, the CompMol_Thermo pipeline embarks on a new field of bio-informed machine learning using metabolic networks and simulated data as constraints for the calculations.
“This is just the beginning,” states Kumar, “It’s a proof of concept to show that computationally-derived thermodynamics values can match experimental values. In the future, we will expand on this by using AI and machine learning to calculate thermodynamic parameters of more complex systems, and eventually be able to predict values without the need for experimental confirmation.”