Computing, Analytics, and Modeling
New PeakDecoder workflow identifies metabolites like never before
Metabolite algorithm developed by computational scientist at Environmental Molecular Sciences Laboratory
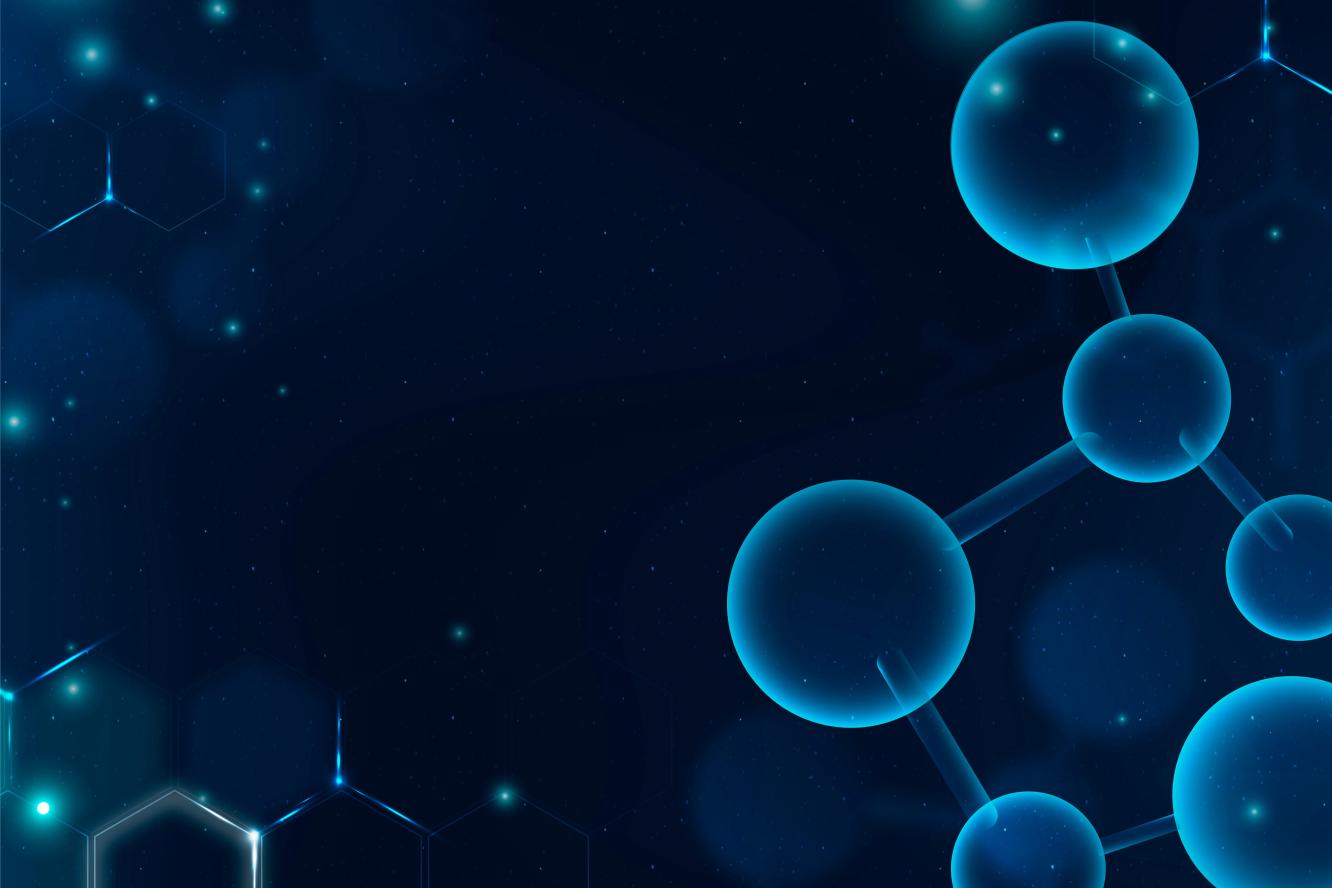
Through PeakDecoder, scientists can now access a new program developed by EMSL computational scientist Aivett Bilbao to identify a range of metabolites using a combination of state-of-the-art scientific instruments and a new algorithm. (Image courtesy of FreePik)
Updated June 6, 2023
Metabolites are basic compounds that sustain life. These small molecules resulting from metabolic processes of cells and biochemical reactions in living systems allow plants and other organisms to grow and thrive by serving as an energy source, signal, or catalyst for important processes.
Identifying metabolites, however, can be challenging due to their complex chemical makeup. They are also vastly diverse, as they are represented in many different classes and structures.
As such, a computational scientist at the Environmental Molecular Sciences Laboratory (EMSL) has developed a machine learning-based algorithm that, combined with modern instrumentation including mass spectrometry, ion mobility spectrometry, and liquid chromatography, can identity many more metabolites in a given sample with a higher level of confidence than ever before. The program is called PeakDecoder.
Aivett Bilbao, creator of PeakDecoder and computational scientist at EMSL, said the new program is a game changer in the world of metabolite identification because it can accurately exploit multiple dimensions: accurate mass, retention time, collision-cross section, and fragmentation patterns from data-independent acquisition mass spectrometry. It is also open source, meaning it is free and readily available for the public to access and use.
“There was no standard method to do this in metabolomics before,” she said. “We are excited because it is a new way to process data and extract information, and we expect it to bring many new scientific discoveries. We can explore data in ways that weren’t possible before.”
A study highlighting the success of PeakDecoder in identifying metabolites with a high level of confidence was recently published in Nature Communications.
How PeakDecoder is different
A range of platforms exist that help to identify and analyze metabolic species. One that is most popular for complex mixtures is mass spectrometry combined with liquid chromatography or gas chromatography separations. However, the many thousands of primary and secondary metabolites in nature entail a high degree of structural diversity that creates significant analytic challenges for detection and annotation using current methods.
Many currently available methods for metabolite identification leave holes in data or result in ambiguous annotations. Some platforms and techniques are great at identifying some types of metabolites, but not others. Others that use machine learning technology are typically limited to estimating confidence of metabolite annotation because rules to globally describe fragmentation mechanisms of metabolites are non-existent. These kinds of rules are routinely used to identify other molecules like proteins and peptides, which are fundamental components of cells that carry out a myriad of important biological functions.
PeakDecoder, combined with other scientific tools, automatically calculates error rates for metabolite identification to enable a sensitive, high-throughput analytical and computational workflow for metabolite identification and accurate profiling. Most current techniques require large libraries as a base for detection with estimated error rates included in the identification. The machine learning training in PeakDecoder works independently of existing spectral annotations or libraries.
“The way I designed it, once the model is trained on the data, the users can interrogate the same raw data, obtaining a number and error metrics that can help them estimate how confident they can be about the presence of a true and high-quality signal for a specific metabolite,” Bilbao said. “The scoring becomes metabolite-centric and libraries of potentially any size can be scored.”
Bilbao said the program uses a new approach that combines machine learning and trains on raw data to calculate the false discovery rate.
“It’s very exciting as it’s a challenge we have been trying to solve for many years,” she said.
Establishing PeakDecoder's success from recent study
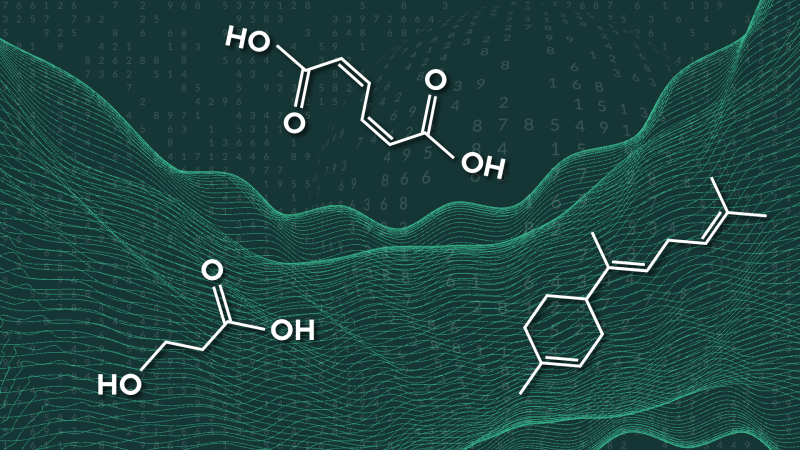
As part of a recent study with the Department of Energy Agile BioFoundry, Bilbao and a team of researchers at EMSL, including Kristin Burnum-Johnson, biochemist and Agile BioFoundry test task lead, and chemist Nathalie Munoz, used PeakDecoder to study 64 metabolites from several fungal and bacteria strains, enabling the interpretation of 2,683 metabolite features across 116 microbial samples. The analyzed strains have been shown to be relevant microorganisms in the biotechnology field for production of value-added chemicals, including biofuels.
Bilbao said their recent success exemplifies the program’s potential for unlocking fundamental information and characteristics of systems that are vital in developing next-generation materials and bioproducts. It could accelerate scientific advancements in the biofuels sector that will help reduce dependence on fossil fuels.
Additionally, Bilbao said the PeakDecoder program unlocks the potential to use predicted libraries for comprehensive metabolite identification, which will help to support a wide variety of applications and industries.
“The metabolomics field is limited by availability of standard compounds,” she said. “You need to generate a library from standards with information that you match against your own unknown samples, but there are so many metabolites that no one has a standard for. PeakDecoder presents an opportunity to use generated predictions from structures of possible metabolites. We can generate predicted libraries using machine learning and then use it to interrogate complex data. That really opens possibilities in environmental science research.”
The future is automation
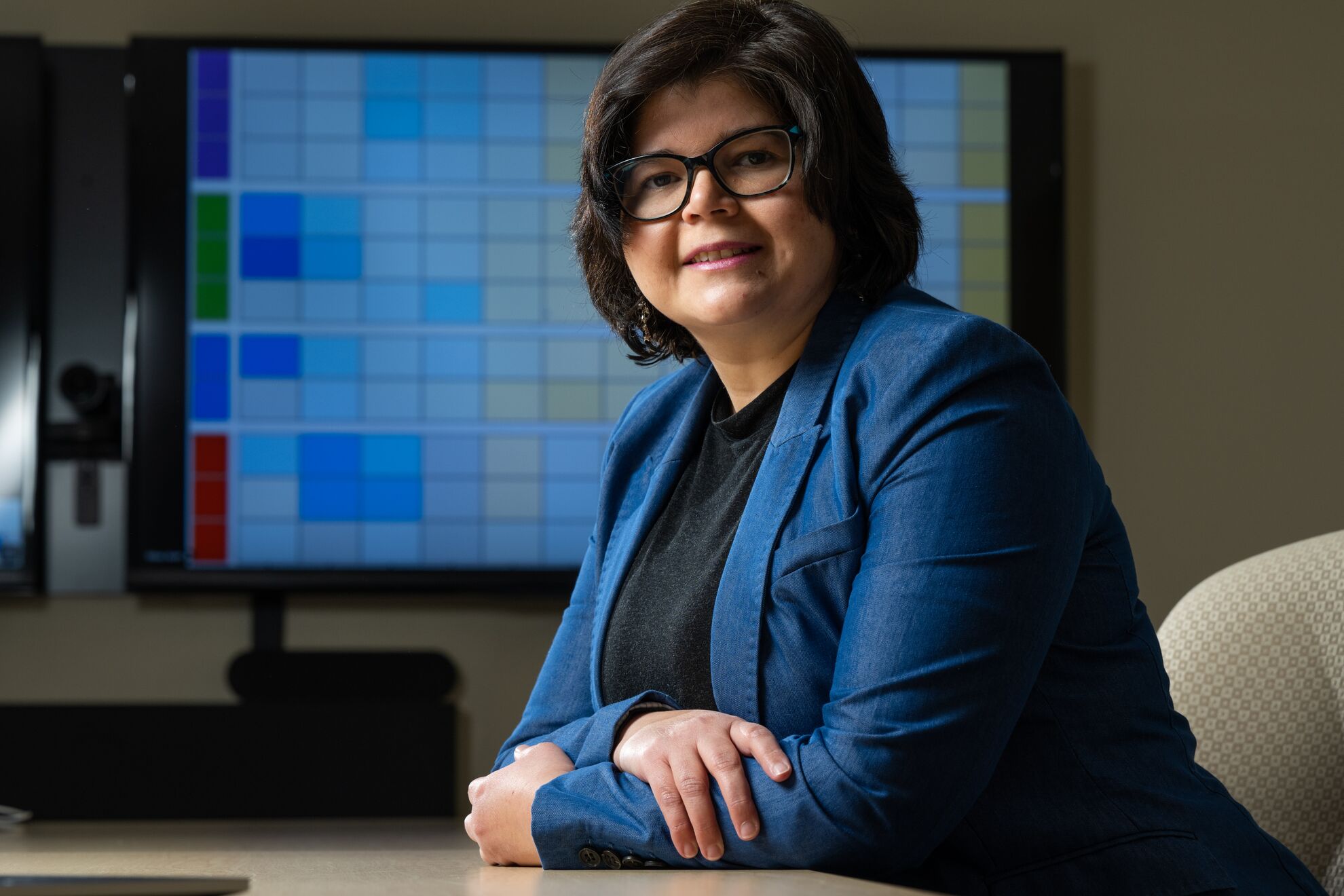
Throughout the next year, Bilbao will be working to make PeakDecoder fully automated. It was built in coordination with several mass spectrometry tools, requiring a series of manual steps. But she envisions that replacing traditional tools with advanced artificial intelligence methods will make the program better and more usable for many scientists.
“The goal is to really make it user friendly so other people can run it very easily with their data,” she said. “In coordination with respective EMSL research areas, PeakDecoder is enabling essential data transformation for mass spectrometry because the files that come out of the instrument are translated to a list of molecules that can be used by scientists for biological interpretation. That will enable research advancements not only at EMSL with our own scientists and users, but also with the larger scientific community.”
For more information on PeakDecoder, contact Bilbao at aivett.bilbao@pnnl.gov. The PeakDecoder program is currently available to access via GitHub.