Computing, Analytics, and Modeling
New Algorithm Identifies Metabolites Using Machine Learning
Metabolite profiling advances to a new level of sophistication.
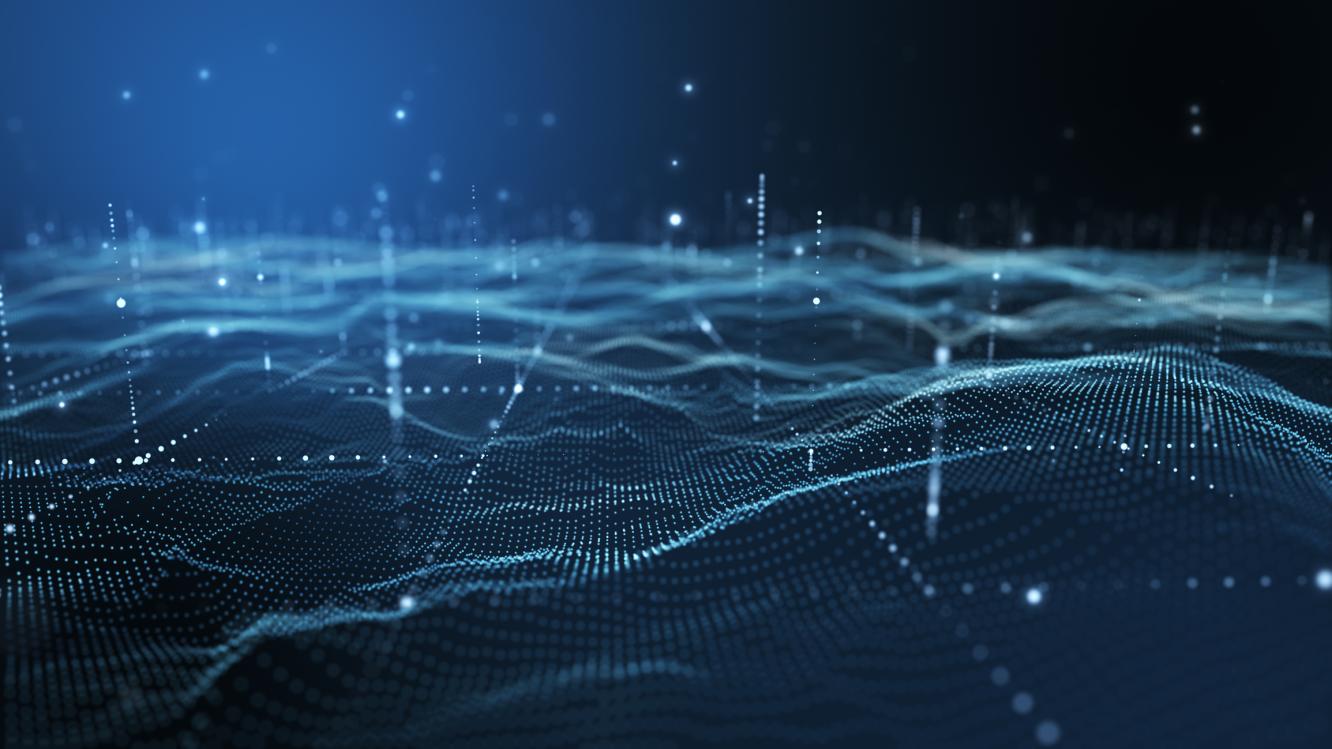
In a publication highlighting PeakDecoder, a multi-institutional team of researchers optimized a combination of sophisticated instruments and crafted the machine learning algorithm to analyze metabolomics data resulting from it. (Photo by Andrea Starr | Pacific Northwest National Laboratory)
The Science
In a recent study, a multi-institutional team of researchers demonstrated a new high-throughput and accurate workflow to identify metabolites. They combined liquid chromatography, ion mobility, and mass spectrometry instrumentation to collect data and analyze the metabolomes of fungi, bacteria, and yeast. Then, they used machine learning within a newly crafted algorithm, called PeakDecoder, to confidently identify and quantify metabolites in samples from these various types of microorganisms.
The Impact
The research team established a multidimensional library of 64 metabolites of interest for the microorganisms they studied, all of which are relevant in the biotechnology field for producing value-added chemicals. Using PeakDecoder, researchers can now very accurately identify these and other metabolites of interest from other fungi, bacteria, and yeast. Once researchers better understand the metabolomic landscape in these various types of microorganisms, they can re-engineer the microbes to produce bioproducts for environmentally friendly industrial applications.
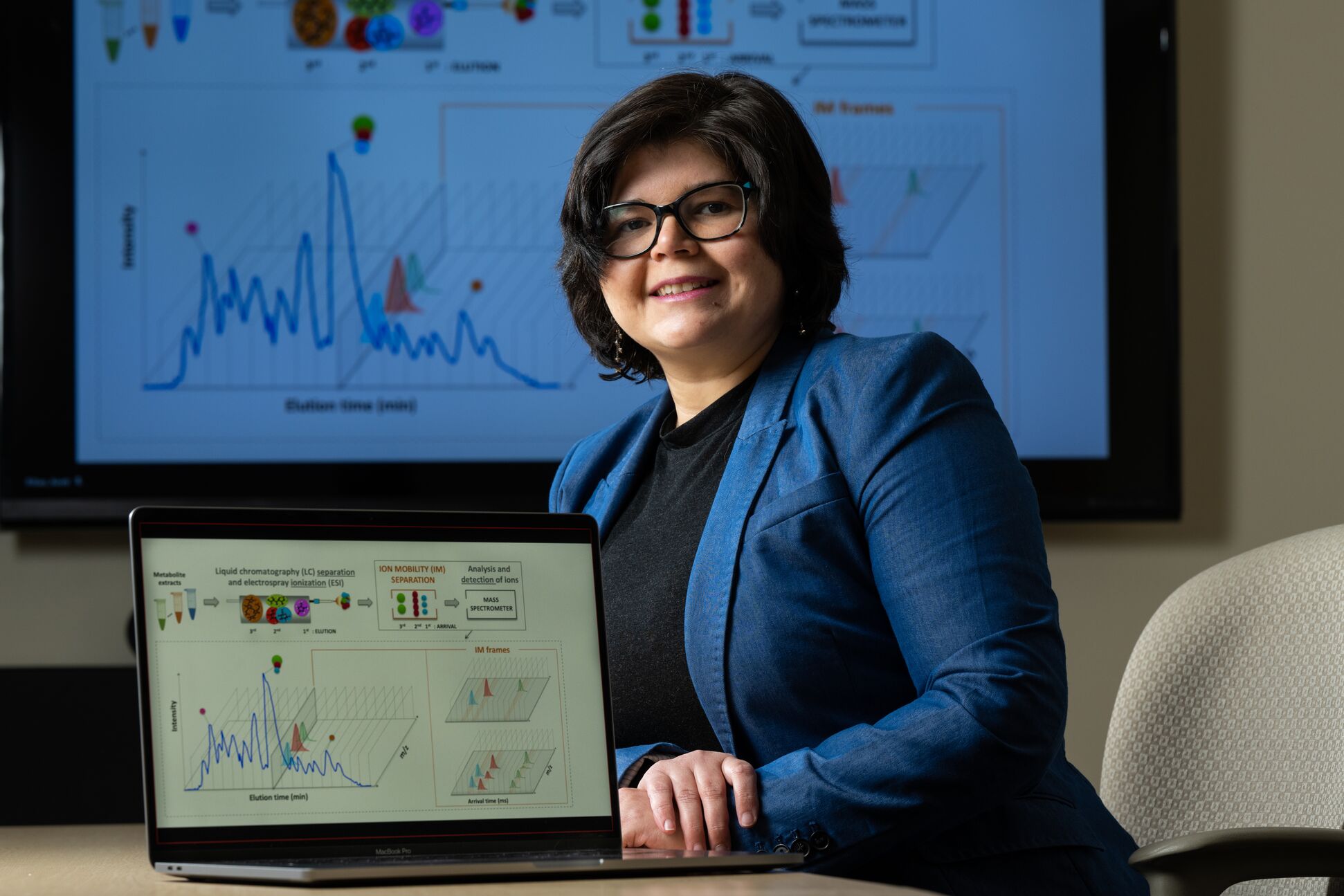
Summary
Metabolites, key actors in the biochemical transformations that occur within and across living organisms, are small and chemically complex molecules. The vast diversity of metabolites—different classes and structures—make identification challenging. Furthermore, rules to describe fragmentation mechanisms of metabolites, which are used to identify other molecules like peptides, are nonexistent. For these reasons, the confident identification of the components of metabolomics has remained elusive, with methods for controlling error rates. Until now.
A computational scientist at the Environmental Molecular Sciences Laboratory, a Department of Energy (DOE) Office of Science user facility located at Pacific Northwest National Laboratory in Richland, Washington, has created a new algorithm called PeakDecoder, which can identify individual molecules in complex mixtures. In a publication highlighting this accomplishment, a multi-institutional team of researchers optimized a combination of sophisticated instruments and crafted the machine learning algorithm to analyze metabolomics data resulting from it. The potential for this kind of data has been previously reported, but algorithms to fully process these data were still needed. PeakDecoder helps distinguish individual metabolites from signals of combined metabolites and calculates errors in metabolite identification, thus enabling accurate metabolite profiling.
Armed with this tool, researchers can build more comprehensive metabolomic landscapes for specific types of microorganisms. As they understand more about each metabolite within a microorganism, they can manipulate the metabolite production for manufacturing value-added chemicals.
The next step for advancing PeakDecoder is to leverage state-of-the-art artificial intelligence methods to enable the algorithm to become automated for ease of use and for application in other types of molecular profiling research, including proteomics and lipidomics research.
Contacts
Aivett Bilbao, EMSL, aivett.bilbao@pnnl.gov
Kristin Burnum-Johnson, EMSL, kristin.burnum-johnson@pnnl.gov
Funding
This work was part of the DOE Agile BioFoundry, which is supported by DOE’s Office of Energy Efficiency and Renewable Energy, Bioenergy Technologies Office, and used capabilities developed under a National Institute of General Medical Sciences grant. Microbial biomass samples from fermentations on different hydrolysates were generated as part of the Feedstock Conversion Interface Consortium, funded by DOE’s Bioenergy Technologies Office. Portions of this research were performed at the Environmental Molecular Sciences Laboratory (EMSL), a DOE Office of Science user facility sponsored by the Biological and Environmental Research program.
Publication
Bilbao A., et al., "PeakDecoder enables machine learning-based metabolite annotation and accurate profiling in multidimensional mass spectrometry measurements." Nature Communications 14, 2461 (2023). [DOI: https://doi.org/10.1038/s41467-023-37031-9].